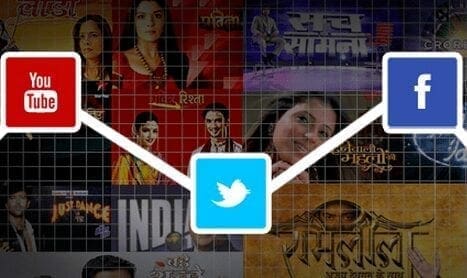
Six months ago, on Facebook I had done a survey. The question posed was “Which is your favorite Hindi film scene?”. I have analyzed the data and I present to you my analyses and my interpretation of this analyses. An example of how profiling can be done based on social media data analytics. Also an example of bad statistical science.
1. I got 26 people who responded. Total number of friends that I have is 855. This implies that probability of people liking Hindi films amongst my Facebook friends = 26/855 = 3%. Is this a good estimate of number of people in India who like Hindi films? Is it an underestimate or overestimate?
To answer this let us assume that all people who like Hindi films saw Chennai Express which has done a business of Rs 150 crores. Assuming average ticket price of Rs 75, this implies that 20 million people saw it. Assuming a population of 1.25 billion, this implies that proportion of folks who like Hindi films is 20 million/1.25 billion = 2%. So in my friend’s circle I can assume that the I am hanging out with Facebook folks who like Hindi films more than the average Indian and I am happy about this. The theory behind this can also be easily explained – both me and my wife are big Hindi film fans, so it is natural that we hang out with like-minded folks.
2. I got 6 female responders and 20 male responders. So the number of female friends is 6/26 which is 23%. I was depressed as this too low. Is this an underestimate or an overestimate? To check, I randomly sampled 176 of my friends which is the required sample size to estimate the truth (assuming it to be 50%) with a precision of 5% for a 90% confidence interval. When I did the gender mapping of these 176 folks, I found 70 women and 106 men for an estimate of female Facebook friends to be 40%, which I am much more comfortable with. So what does 6/26 or 23% represent. It represents female Facebook friends who like Hindi films.
This implies that among my Facebook friends who like Hindi films, ¼ are women and ¾ are men. So there are two interpretations possible – women like Hindi films less than men or among the people I hang out with, I hang out with men who like Hindi films and women who do not like Hindi films! Hmmm… Need to think this through.
3. Multiple scenes per person as a choice. Amongst 20 men, there were 59 scenes that were listed as favorites for an average of 3 scenes per person. For women, they listed 20 scenes among 6 of them, indicating an average of 3 scenes per person. But out of 20 men, there were 8 men who listed only one scene whole among 6 women 3 listed a single scene.
This indicates that while average number of favorite scenes was same, females were much more sure about their choices then were males. As usual men are confused. So now I am Facebook hanging out with men, who love hindi movies but are confused.
4. I also classified my 176 friends and 26 friends who responded into old and young based on my judgment (sorry if I got it incorrect). Amongst 26 responders, 4 were old and 22 were young. But when I compared this with the sample of 176, I found that 68 were old out of 176 indicating that old men did not like Hindi films or do not hang out in Facebook with me.
With this analyses, I have concluded that I am hanging out with men, who love Hindi films, are confused and are young. A good profile is being created for me.
5. Finally the result of the poll. Most popular film – Dabaang and Sholay followed by Deewar, Anand and Jaane Bhi Do Yaaron.
Isn’t it amazing. I would have picked these films myself without the polls. I am an old-timer, so Dabaang did not feature for me, but since I hang out with young men, it is not surprising that Dabaang made it to the list.
6. The most popular star was Salman Khan, followed by Amitabh Bachhan and next Sharukh Khan.
Is this amazing or what? The three biggest stars came out even in this small sample. There is a definite method behind the big data analytics madness that is going on.
Final conclusion – For Ashwini Mathur – promote things which young men like, related to Hindi movies, promoted by or related to Salman, Amitabh or Sharukh. Since he is confused never promote anything very strongly. Give choices.
An example of what not to do if you are a statistician – making theory up as you go along finding statistical results.
About the Author: Ashwini Mathur is a resident of Hyderabad. In the past he has worked at GSK Pharmaceuticals as a Senior General Manager and at Novartis after that. He has a Master’s degree in Mathematics from IIT Delhi, a PhD in bio-statistics from University and College Berkeley, California and an executive MBA from IIM Bangalore.
Be the first to comment